Using the same substrate to make different imaginary maps
Exploring maplike determinism with Processing and Axidraw
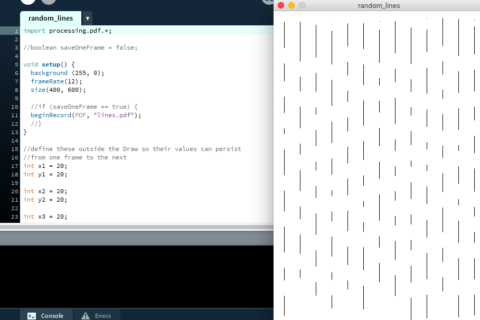
Using the same substrate to make different imaginary maps
The recent months have been full of Christmas card design projects. For this card I was tasked with creating a design based on the same influences I’m grappling with in my personal work, and I went in quite an experimental direction. My brief to myself was to create something in the same mode as my Central […]
Project incorporating weather data to dictate form
Project focusing on making drawings limited by randomly-chosen values
One of my many goals this year has been to learn more about scripting and procedurally generating graphics, for which I’ve been using the language Processing. I don’t have a lot of prior experience with code, though, and that makes things a bit difficult! It’s a totally different way of thinking. Processes that seem simple enough […]